Untamed: Unconstrained Tensor Decomposition
and Graph Node Embedding for Cortical Parcellation
- 1Ming Hsieh Department of Electrical and Computer Engineering, University of Southern California
- 2Athinoula A. Martinos Center for Biomedical Imaging, Massachusetts General Hospital and Harvard Medical School
- 3Center for Neurotechnology and Neurorecovery, Department of Neurology, Massachusetts General Hospital and Harvard Medical School
- 4Radiology and Pediatrics, Division of Neonatology, Children's Hospital Los Angeles
- 5Keck School of Medicine, University of Southern California
Introduction
Untamed is a fully automated framework for cortical parcellation that bridges large-scale network identification and region-level parcellation from resting-state fMRI. It integrates unconstrained tensor decomposition via NASCAR, without relying on strong assumptions like statistical independence, with graph-based node embeddings to generate spatially coherent, functionally homogeneous cortical regions. Compared to existing atlases, Untamed delivers superior or comparable performance in functional connectivity homogeneity and task alignment across multiple datasets.
Framework
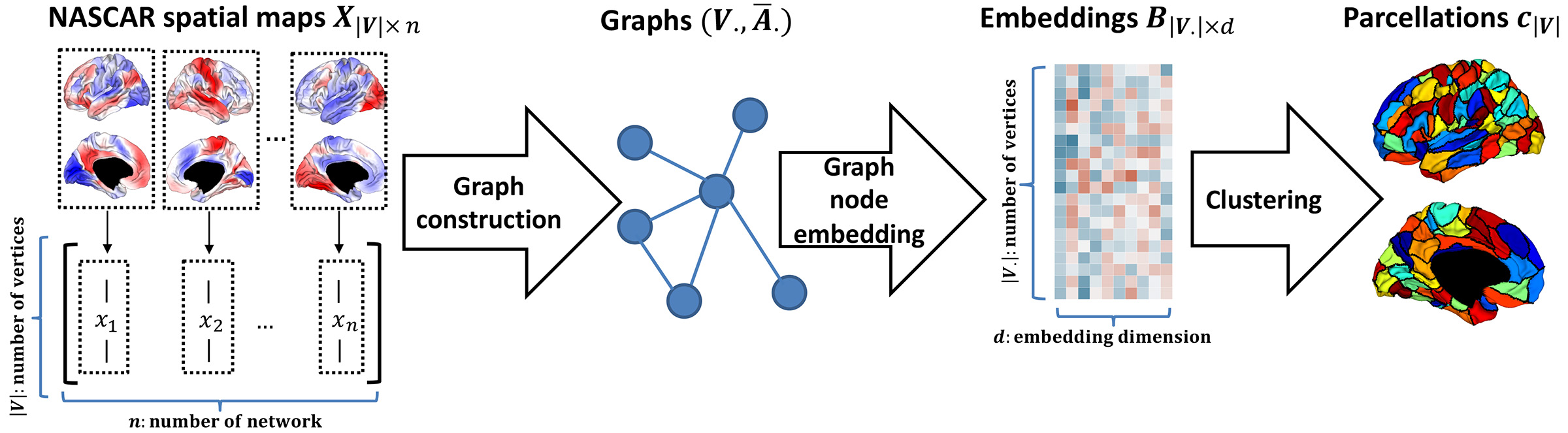
Performance
Resting-state functional connectivity (RSFC)homogeneity
Note: Higher values indicate better homogeneity
Weighted average RSFC homogeneity on the Yale / HCP dataset. Each bar plot depicts the subject-wise RSFC weighted average homogeneity, averaged across test subjects, for each baseline and the Untamed atlas, with matched parcel numbers for the left and right hemispheres. The error bars represent the standard error across all subjects.
Task contrast alignment
Note: Lower values indicate better alignment
Weighted average task contrast variance evaluated on MDTB / HCP test subjects. Each violin plot depicts the alignment with task contrast maps, computed for the baseline and the Untamed atlas, with matched parcel numbers for the left and right hemispheres.
Key ablation study
Input spatial feature map: NASCAR vs ICA
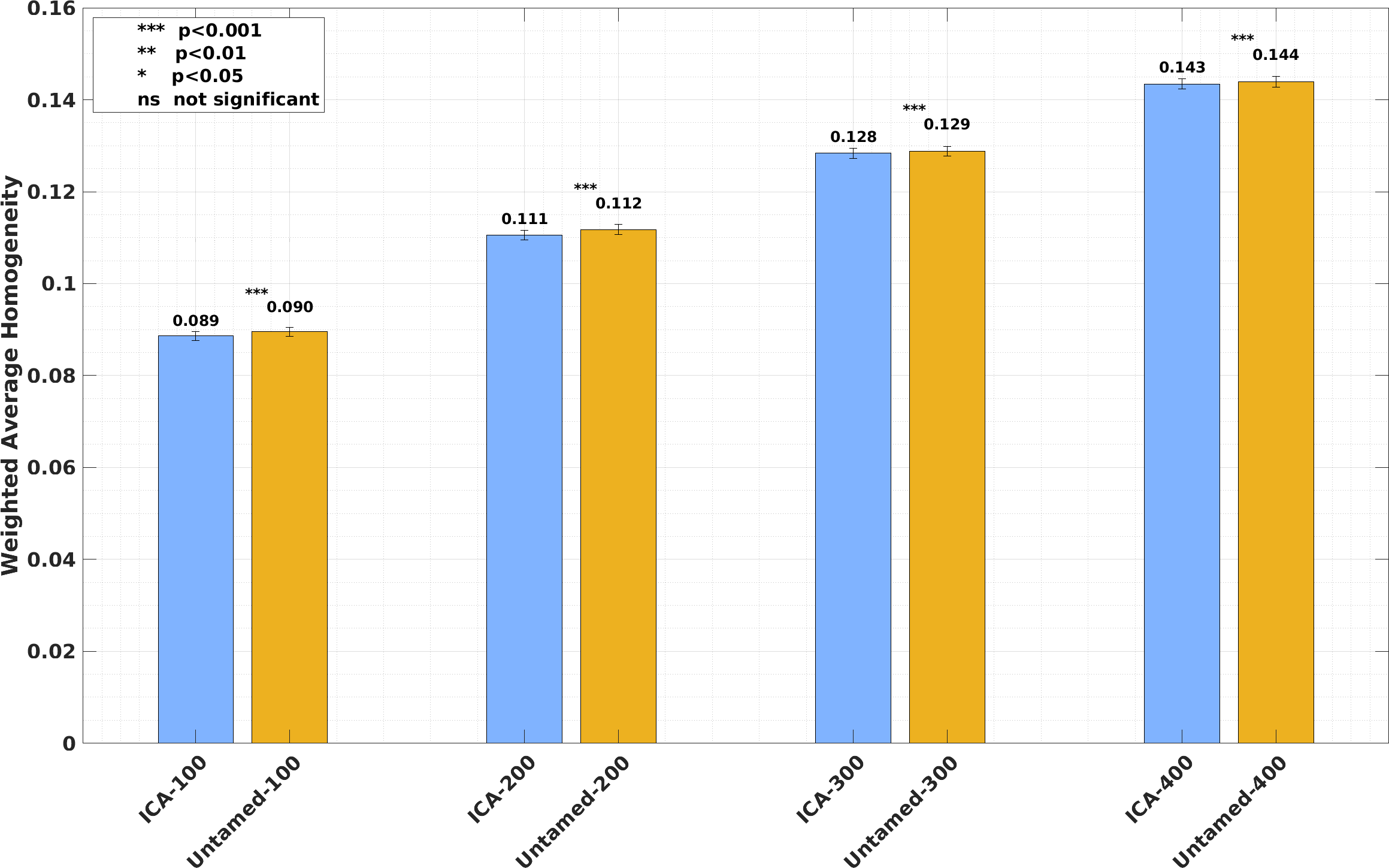
Weighted average RSFC homogeneity on the HCP dataset. Each bar plot depicts the subject-wise RSFC weighted average homogeneity, averaged across test subjects, for each atlas generated from ICA maps and the Untamed atlas (generated from NASCAR maps). Parcel numbers matched for the left and right hemispheres. The error bars represent the standard error across all subjects. The ICA baselines, derived from and optimized through hyperparameter tuning on the same HCP dataset, benefit from this dataset-specific tuning. In contrast, the Untamed atlas was generated using the independent GSP dataset with hyperparameters tuned on GSP. The ICA results represent the maximum homogeneity achievable under optimal hyperparameter settings for the HCP dataset.